Introduction - GPU Ads (NAUSEAM)
If you have a title such as CIO, CTO, or one even loosely related to AI/ML you’ve probably been served ads touting AI Clouds with the latest and greatest GPUs (perhaps even by us.) In a relatively new space, marketing teams are scrambling to get your attention with promises of high-performance hardware. It makes sense, NVIDIA is the center of gravity for high-performance AI/ML compute and having availability of their cutting edge hardware is a solid selling point.
As the industry continues to evolve and advancements in hardware continue to push the boundaries of what is possible even the best hardware can underperform when it’s not supported by a great hardware stack. In the Olympics one person can win the gold in wrestling but it takes an amazing team, working together, and complimenting each other’s skills to win the gold in the team competitions.
AI clouds are more akin to team sports than they are to wrestling. A GPU alone can’t make your models train faster but a GPU with the right solutions supporting it can. This guide is intended to help companies make the right choice when it comes to the performance of their GPU Cloud provider. Whether you are looking to invest to support internal, commercial, and/or mission critical AI solutions, understanding the variables that contribute to a high performing cluster will help you select the cloud that will effectively support your teams today and into the future.
In this guide we cover three key factors to consider when evaluating the performance of a GPU Cloud:
- Access to Cutting-Edge Hardware
- Consistent Performance and Reliability
- Scalability and Future-Proofing
Access to cutting-edge hardware
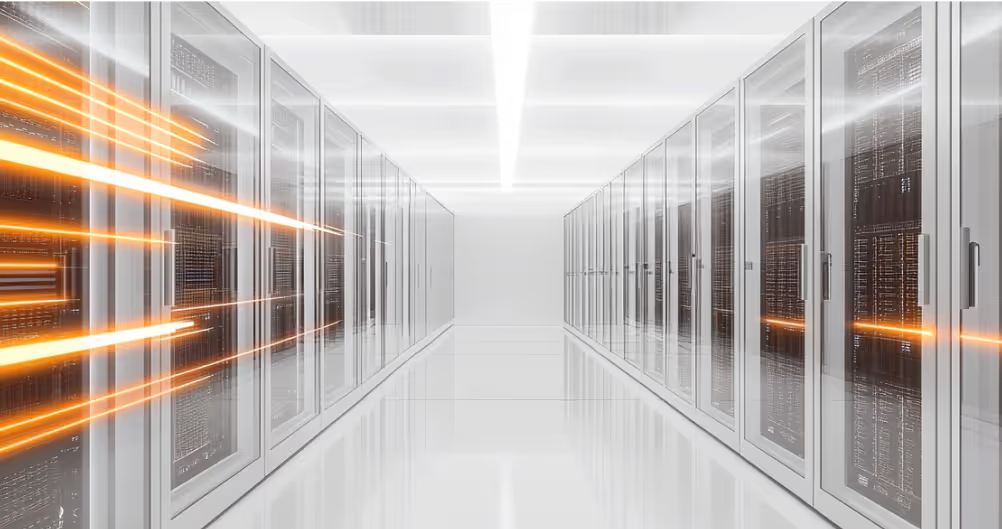
AI workloads evolve fast. While innovations such as DeepSeek R1 promise great outcomes with lesser hardware, the truth is that as your models evolve and advance, datasets grow, and demand increases so too will your need for compute capacity. At the same time hardware is evolving and advancing and both you and your competitors will be looking for an advantage. The most obvious advantage is having access to the latest, most powerful, GPUs.
When selecting a vendor, understanding their ability to acquire and deploy cutting edge hardware is critical. The vendor must scale with your business and give you the opportunity to gain a competitive advantage. Vendors who lag in this category may ultimately hold your teams back and prevent innovation that might catapult your business.
- Does the vendor offer the latest GPUs as soon as they become available?
- Is there a clear pathway for migration to updated hardware from a technical and pricing perspective?
- How quickly can they scale GPU capacity to match your growth?
- Is the infrastructure purpose-built for AI, or is it a repurposed general cloud?
Consistent Performance and Reliability
.avif)
Many providers market high-performance compute, but inconsistent performance, slow data access, and unexpected downtime can cripple training efficiency. A chain is only as strong as its weakest link and any AI cluster is only as fast as the slowest part of the stack. Performance restrictions can slow innovation and reduce the value of your AI investments. Downtime, on the other hand cuts directly into your bottom line with Gartner estimating that server downtime costs, on average, $5,600 per minute.
Gartner estimates server downtime costs
Many new entrants in this space are re-purposing other hardware with shiny new GPUs to capitalize on the growing market demand. This might be the right solution for some buyers however these solutions often overlook the other critical parts of the stack including data transfer, ISP and power redundancy, storage configurations, and CPU design. Any of these variables can have a significant impact on the performance and efficiency of your AI cluster.
Understanding how the infrastructure was designed, the expertise behind the design, and what hardware was selected to complement the GPUs will help ensure you are selecting a solution that will live up to the demands of your workloads.
- Is the infrastructure purpose-built for AI, or is it a repurposed general cloud?
- What storage and networking optimizations exist to reduce training latency?
- Can the vendor provide performance consistency, not just peak specs? Can they show reports from their monitoring platform to prove their claims?
- How do they handle failures, redundancy, and uptime commitments?
Scalability & Future-Proofing
AI model sizes are growing exponentially. A solution that works today but can’t scale seamlessly will force painful migrations later. The answer may be you’ll want to move to a larger cluster and are prepared for that eventuality. However, over time you may have a need for distributed workloads across clusters or even data-centers. You may have unique data storage requirements that prevent a centralized AI workload and require increased throughput to support performance. Whatever the future looks like for your business, it's important to evaluate your vendor’s ability to adapt and meet your needs as they evolve.
- Can the platform scale dynamically with your needs?
- Does the vendor provide high-speed interconnects for distributed training?
- Is there flexibility on how workloads are deployed and expanded?