For companies that aren’t AI native, who by their very existence are focused on AI, the adoption of AI is more about ‘when’ than ‘if’. For these companies the adoption of AI will ultimately have a significant impact on their business but getting started is likely to be more disruptive. Determining where to focus, finding the right talent, building and training models, sourcing hardware infrastructure, and training employees are all pieces of the puzzle that need to be considered. As a result, AI adoption in the traditional enterprise tends to follow a more cautious and pragmatic path.
As teams define and execute their strategy, one of the key considerations will be around how to source the hardware necessary to support their mission critical workloads. While infrastructure decisions often start small, the lifecycle of an AI project—especially in highly regulated fields like life sciences—demands flexibility, foresight, and ongoing adjustment.
In this blog post we will discuss infrastructure in the context of the AI lifecycle focusing on key considerations that influence a decision around hardware sourcing and deployment.
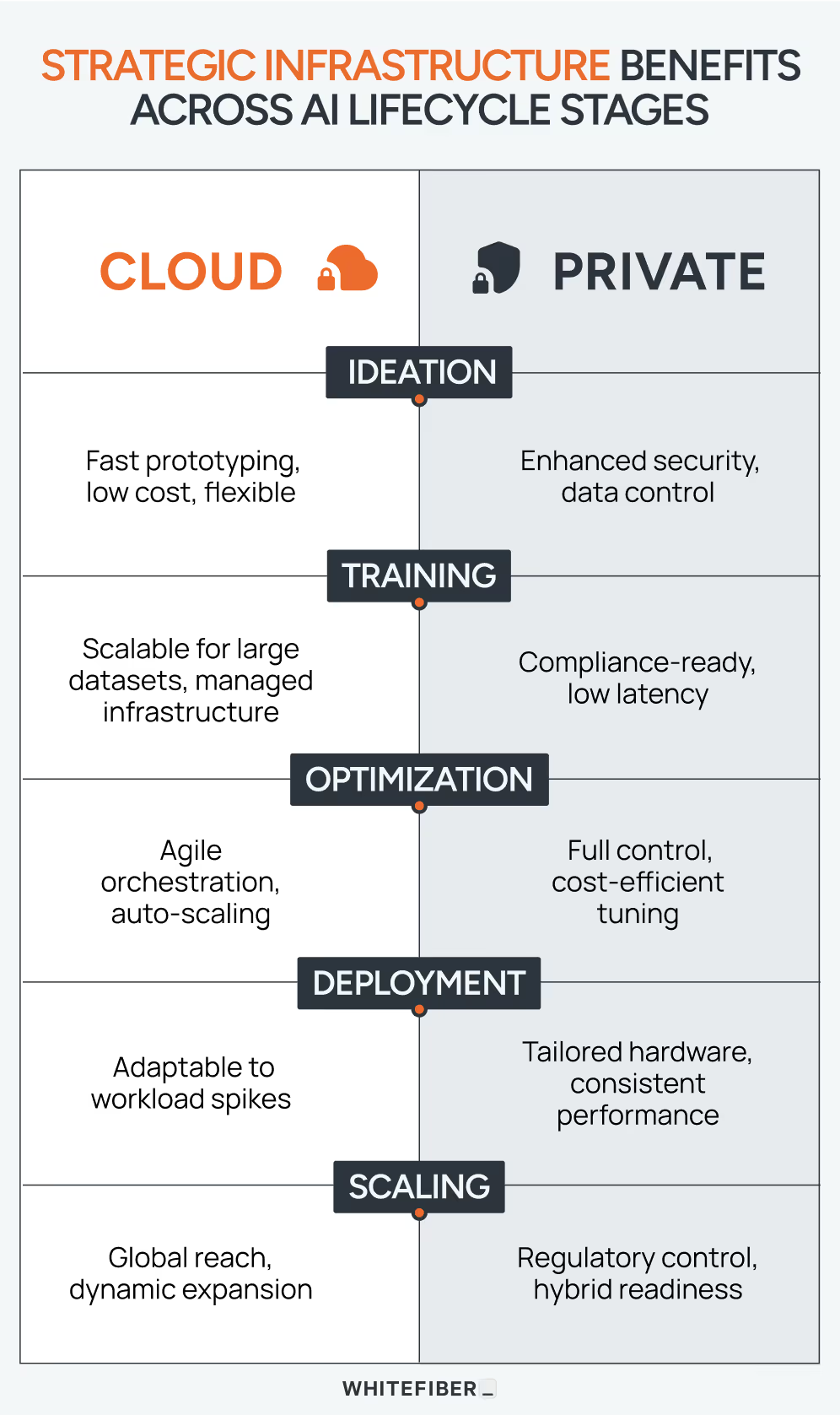
1. Ideation & Prototyping: Building the Foundation
When kicking off a project infrastructure needs are often straightforward. The primary goal is rapid prototyping and validation of AI concepts. At this stage, modest cloud-based GPU infrastructure—leveraging versatile resources like NVIDIA H100 GPUs allow teams to quickly iterate models with minimal upfront costs.
Starting with scalable cloud solutions avoids heavy initial investments, offering flexibility as projects evolve. Prioritizing flexibility and rapid iteration in early stages to validate ideas efficiently.
2. Model Training & Fine-Tuning: Performance at Scale
After successfully validating the initial prototype, additional resources (sometimes substantially more) are required to train and refine models using extensive datasets. At this point transitioning to high-performance computing environments, deploying advanced GPUs like NVIDIA H200 and B200 and leveraging high-speed, low-latency storage solutions from WEKA and VAST Data is common. At this point, the choice between cloud or private AI infrastructure starts to become more important, particularly for teams in highly regulated environments. Geographic factors, data residency compliance, and other government and industry regulations can heavily weigh on the outcome of a decision about whether or not to continue investing in cloud resources.
Cloud vs. Private Infrastructure:
Strategic investments in high-performance hardware and optimized storage during training accelerate model readiness, ultimately reducing time-to-value despite increased initial expenditure. Ensure that the cloud providers you are evaluating meet the standards for security and compliance that your business must adhere to and ensure they can scale geographically with your business.
In many cases colocation of infrastructure makes the most sense as it provides the opportunity to control for these factors. Understanding the capacity of your infrastructure relative to your scaling plans is critical. This will allow you to proactively plan for growth and limit hardware availability’s impact on your growth. Evaluating your data center partner’s ability to help with procurement and set-up today will help avoid surprises down the road.
3. Iteration & Optimization: Accelerating Through Efficiency
At the optimization stage, infrastructure needs shifted towards efficiency and agility. Implementing solutions such as Kubernetes orchestration to manage resources dynamically, enabling rapid testing and iteration cycles. Infrastructure monitoring becomes essential, providing insights to streamline compute utilization and minimize waste.
Cloud vs. Private Infrastructure:
Efficient orchestration and resource monitoring tools helps avoid overspending by optimizing resource usage, a critical cost-saving measure during iterative refinement. Cloud vendors often offer solutions for this as part of their services. Ensuring that these tools work seamlessly with your DevOps toolchain and existing observability solutions is important to ensure your workflows are not impacted and that your teams have a lower learning curve for managing these new workloads.
4. Inference & Deployment at Scale: Precision and Responsiveness
Deploying AI platforms for real-world use, raises new challenges. Inference workloads require lower latency, high reliability, and often heterogeneous compute environments. By deploying a mix of NVIDIA B200s, H200s, and H100s GPUs tailored to specific model requirements, teams can achieve balanced performance, efficiency, and cost-effectiveness.
Cloud vs. Private Infrastructure:
Smart resource diversification for inference workloads minimizes unnecessary spending, allowing teams to optimize cost-efficiency at scale. Ensure your cloud vendor can support heterogeneous environments and that they are able to secure and deploy next gen hardware consistently and reliably.
If owning your infrastructure is the best path, heterogeneous environments can help you extend the value of your investments today. Over time, as new innovations in hardware for AI workloads are released, older generations can be repurposed thus extending their useful lifecycle. While there is much talk about the useful life of a GPU being less than five years, the truth is there are plenty of ten-year old A100s offering significant value thanks to thoughtful repurposing.
5. Enterprise-Grade Scaling in Regulated Environments: Compliance at the Forefront
Scaling across regulated markets brings stringent compliance and auditability requirements. Shifting towards hybrid and private AI environments, combining public cloud agility with private infrastructure security and compliance can be a powerful advantage while simultaneously limiting compliance risk. Organizations that need occasional capacity for times of high compute demand can benefit greatly from colocation environments that allow for dynamic scaling of select resources to ensure consistent performance.
Cloud vs. Private Infrastructure:
Strategic investment in hybrid and private infrastructure ensures compliance and mitigated risks, ultimately offering protection from costly regulatory penalties and disruptions. Factoring the cost of compliance incidents and security breaches should be part of the formula when considering the capital expenditure of procuring and colocating hardware.
Conclusion
AI infrastructure requirements evolve significantly over a project's lifecycle. Anticipating these shifts, or at least recognizing indicators prompting infrastructure changes, is key to managing cost, performance, and compliance effectively.
As the AI Infrastructure Company, WhiteFiber is focused on helping organizations navigate their journey across the AI lifecycle. From our highly performant cloud to custom designed hybrid deployment solutions we aim to be a strategic partner for enterprise adoption of mission critical AI.